December 19, 2024 report
This article has been reviewed according to Science X's editorial process and policies. Editors have highlighted the following attributes while ensuring the content's credibility:
fact-checked
peer-reviewed publication
trusted source
proofread
Machine learning helps researchers develop perovskite solar cells with near-record efficiency
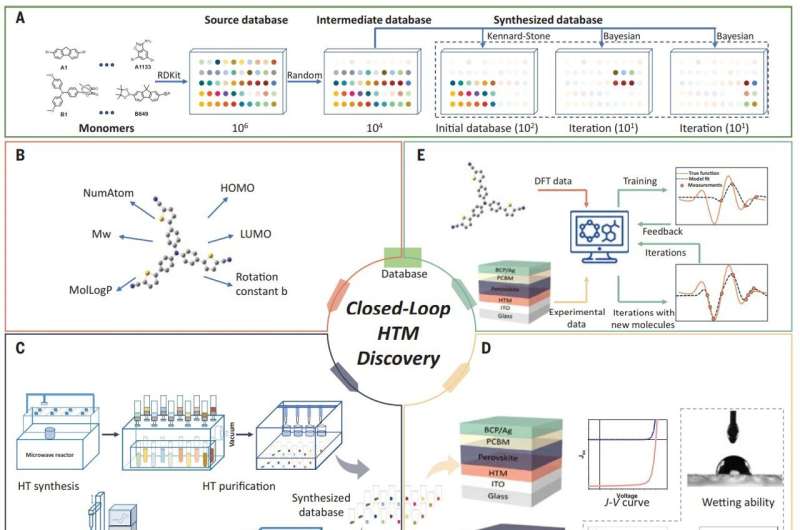
An international team of scientists has used machine learning to help them develop perovskite solar cells with near-record efficiency. In their paper published in the journal Science, the group describes how they used the machine-learning algorithm to help them find new hole-transporting materials to improve the efficiency of perovskite solar cells.
Currently, one part of a solar cell is called the hole-transporting layer. Its purpose is to carry electron-hole pairs generated from a stable electron by a semiconductor after a photon is absorbed. The effectiveness of such transport plays an important role in the efficiency of the solar cell—and its effectiveness is directly associated with the material from which it is made.
Thus far, few have been found that are effective for commercial use. The researchers note that all of them have been discovered via experimentation with existing structures, rather than applying a basic understanding of how they work. In this new effort, the research team has taken a new approach to finding a new effective material using machine learning.
The machine-learning algorithm was facilitated using 101 molecules selected from a dataset of over a million candidates. Test solar cells were made using synthesized materials, the results of which were used as training material for the AI. The algorithm was then asked to come up with promising new material candidates—it replied with the 24 most promising candidates it could find.
The candidates were then synthesized by the team and put into working solar cells for testing. After several rounds of such testing, the research team settled on a hole-transporting material that resulted in the construction of perovskite-based solar cells with efficiencies as high as 26.2%. The record for such cells, the team notes, is 26.7%, which means their efforts came very close to pushing up the boundary efficiency for such cells.
The researchers note that during their testing, they produced several materials that were close to the most effective, suggesting their approach could be used to produce even more candidates, some of which may be capable of pushing efficiencies even higher.
More information: Jianchang Wu et al, Inverse design workflow discovers hole-transport materials tailored for perovskite solar cells, Science (2024). DOI: 10.1126/science.ads0901
© 2024 Science X Network